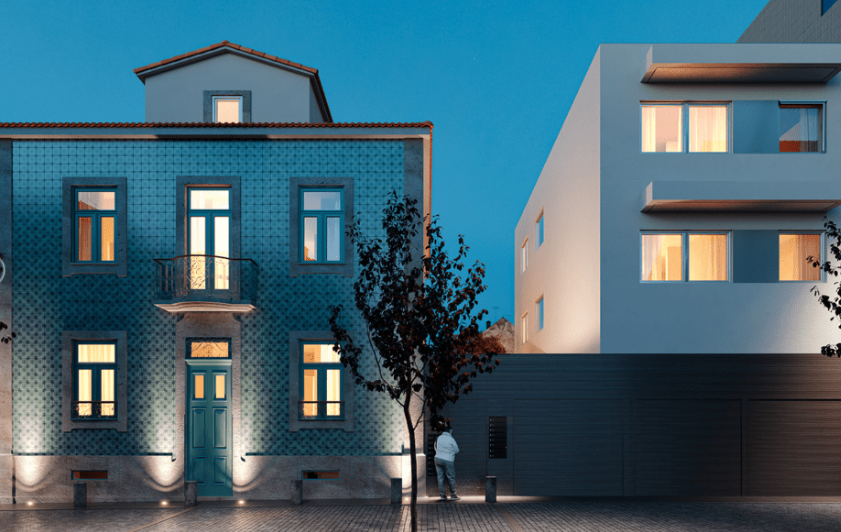
How accurate is AirDNA? I tried it for a month.
I first used AirDNA back in 2019 to find the most profitable London boroughs for my Airbnb Arbitrage business.
- Last updated April 25, 2024
I first used AirDNA back in 2019 to find the most profitable London boroughs for my Airbnb Arbitrage business. I decided to purchase their expensive subscription, which was over $200 (I think). Before going further, this is just my personal story about my experience with AirDNA, its data accuracy, and why I built Airbtics.
I was skeptical about their data accuracy, so I asked a few Airbnb host friends if their income matched what’s shown on AirDNA’s Rentalizer. I got mixed results. Half said it’s similar, and half said it’s far.
My job back then was a software developer at WhatsApp, so as a developer, I started to dig into how they collected the data. By reading their pages, I can tell that they 1) scrape from Airbnb listings and 2) get booking data directly from hosts who have agreed to give them the data. That’s cool.
Then, my question was, if they scrape the data from Airbnb calendars, how can they tell if a listing was booked by a guest or blocked by a host? Because I played with Airbnb’s API, and they do not show whether a night is booked by a guest or blocked by a host. It just shows it as “blocked”. AirDNA says that Airbnb used to show this information back in 2014, and they took advantage of that and built a classifier. That’s interesting.
So, here’s a small tip.
Verify your data using ENEMY method
Along with the calculator, you can also use the ENEMY method to ensure you estimate the accurate amount of revenue. The ENEMY method simply means going to the Airbnb site to find 5-10 listings that are most similar to the house you want to list on Airbnb. Then, check how many days are booked in the next 6-8 weeks. Also, check how many reviews on average they get every month. Compile them on an Excel sheet. You may see some interesting patterns.
Anyway, thanks to AirDNA, I realized Airbnb Arbitrage won’t be that profitable in London. That is lucky to me because the Covid hit hard a few months after I made this conclusion.
My thoughts on AirDNA’s rentalizer
I’ve had a few difficulties using AirDNA. One is that they do not have some markets in Asia and South America where I was interested in buying an investment property. Another problem is their submarket boundary definition. On some occasions, two areas in a submarket have a completely different average occupancy rate. A common example is that, in beach town, properties that are right by the ocean have a higher occupancy than the ones that are located inland. Also, I was not convinced about their approach to data accuracy.
So, I built Airbtics, which is an alternative to AirDNA. It has a tailored region feature where you can draw a shape in any markets to get short-term rental data. It has dynamic data updating feature which you can see the Airbnb statistics of the exact micro area you see on the map. Finally, I’ve improved data accuracy of Airbnb occupancy rate data by following method: By labeling the millions of “review” data. Basically, if a series of blocked nights on Airbnb calendar generates a review in the future, we can label those nights as “confirmed booked”. By doing this, our data accuracy improved by 98%, and we were able to score some deals with big-name hedge funds.